Development of a framework for the prediction of slope stability using machine learning paradigms
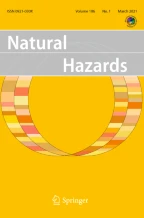
Accurate slope stability prediction is of utmost importance to reduce disastrous effects of slope failures and landslides. However, conventional methods of slope stability analysis are complex and challenging, and more importantly, use of these methods in a wide-area slope stability assessment requires a large number of soil property and field investigation data. These complexities and challenges often demand some simplified statistical slope stability analysis models such as by using machine learning (ML) techniques. So, in this research, we develop slope stability prediction models using multiple linear regression (MLR) and artificial neural network (ANN) and classify the slopes as safe or unsafe using random forest (RF) and support vector machine (SVM) methods. For this purpose, a dataset of 4,208 slope cases was created using limit equilibrium-based Slide software. The effectiveness of each model was then evaluated using statistical metrics and validated through roadside slope cases in Nepal, India, Canada, and the UK. In this study, Spencer’s method-based ANN model was found to have demonstrated the highest reliability. The findings of this work may contribute to simplified and better decision-making process in slope stability assessment, slope safety enhancement, and sustainability improvement in engineering projects involving soil slopes.
This is a preview of subscription content, log in via an institution to check access.
Access this article
Subscribe and save
Springer+ Basic
€32.70 /Month
- Get 10 units per month
- Download Article/Chapter or eBook
- 1 Unit = 1 Article or 1 Chapter
- Cancel anytime
Buy Now
Price includes VAT (France)
Instant access to the full article PDF.
Rent this article via DeepDyve
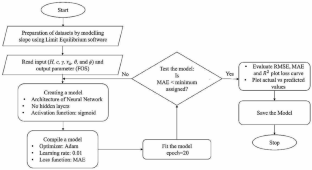
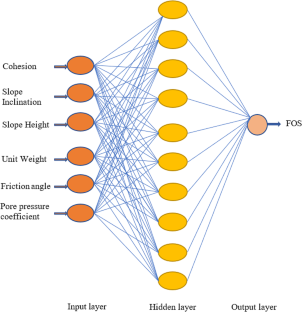
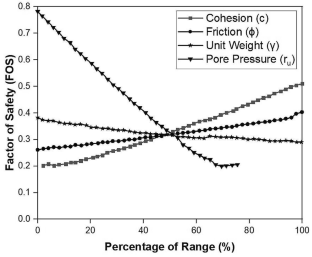
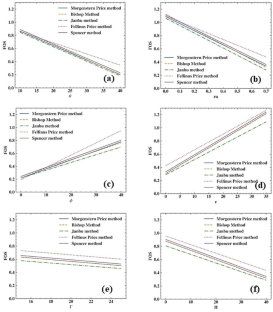
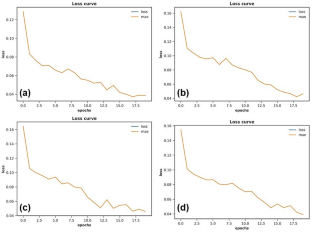
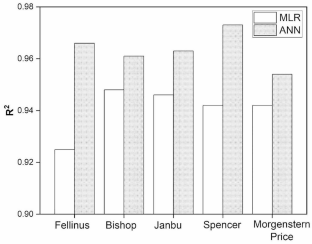
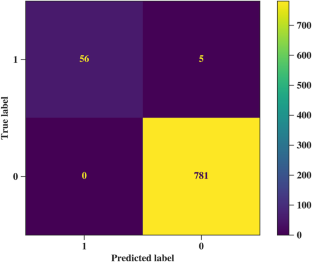
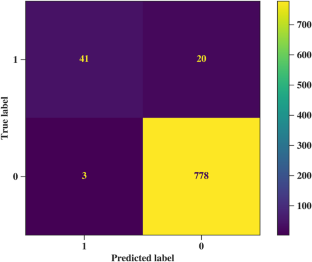
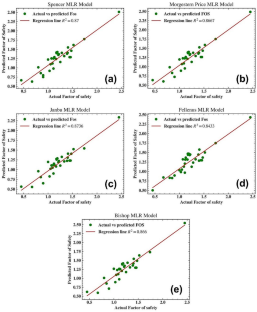
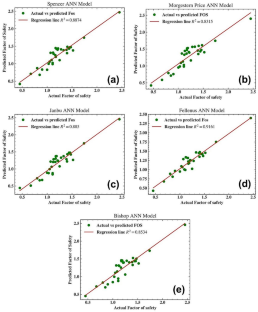
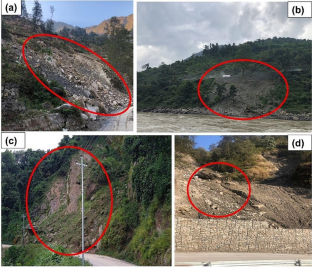
Similar content being viewed by others
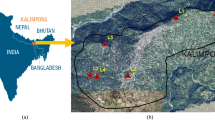
Prophetical Modeling Using Limit Equilibrium Method and Novel Machine Learning Ensemble for Slope Stability Gauging in Kalimpong
Article 23 June 2023
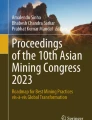
Interpretable Predictions: Machine Learning Approaches to Understand Slope Stability in the Presence of Joint Networks
Chapter © 2023
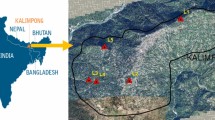
Comparative Analysis of Slope Stability for Kalimpong Region under Dynamic Loading Using Limit Equilibrium Method and Machine Benchmark Learning Classifiers
Article 08 February 2024
Explore related subjects
Data availability
The datasets used and/or analysed during this work are accessible upon reasonable request from the corresponding author.
References
- Abbas JM (2014) Slope stability analysis using numerical method. J Appl Sci 14(9):846–859. https://doi.org/10.3923/jas.2014.846.859ArticleGoogle Scholar
- Acharya IP, Subedi M, KC R (2023) Liquefaction Hazard Assessment of Kathmandu Valley Using Deterministic and Probabilistic Approaches. In Proceedings of the Geo-Risk 2023, Arlington, VA, USA, 23–26; pp. 307–317. https://doi.org/10.1061/9780784484968.03
- Aminpour M, Alaie R, Kardani N, Moridpour S, Nazem M (2022) Slope stability predictions on spatially variable random fields using machine learning surrogate models. ArXiv Preprint ArXiv:2204.06097
- Aryal M, Acharya IP (2022) Machine learning based unified framework for slope stability prediction
- Bansal V, Sarkar R (2024) Comparative Analysis of Slope Stability for Kalimpong Region under Dynamic Loading. Using Limit Equilibrium Method and Machine Benchmark Learning Classifiers
- Bharati AK, Ray A, Khandelwal M, Rai R, Jaiswal A (2022) Stability evaluation of dump slope using artificial neural network and multiple regression. Engineering with Computers 38(Suppl 3):1835–1843. https://doi.org/10.1007/s00366-021-01358-yArticleGoogle Scholar
- Bishop AW (1955) The use of the slip circle in the stability analysis of slopes. Geotechnique 5(1):7–17. https://doi.org/10.1680/geot.1955.5.1.7ArticleGoogle Scholar
- Breiman L (2001) Random forests. Mach Learn 45(1):5–32. https://doi.org/10.1023/A:1010933404324/METRICSArticleGoogle Scholar
- Chakraborty A, Goswami D (2017) Prediction of slope stability using multiple linear regression (MLR) and artificial neural network (ANN). Arab J Geosci 10:1–11. https://doi.org/10.1007/s12517-017-3167-xArticleGoogle Scholar
- Chen Y, Lin H, Cao R, Zhang C (2021) Slope stability analysis considering different contributions of shear strength parameters. Int J Geomech 21(3):04020265. https://doi.org/10.1061/(ASCE)GM.1943-5622.0001937ArticleGoogle Scholar
- Cherkassky V, Mulier FM (2007) Learning from data: concepts, theory, and methods. Wiley
- Cho SE (2009) Probabilistic stability analyses of slopes using the ANN-based response surface. Comput Geotech 36(5):787–797. https://doi.org/10.1016/j.compgeo.2009.01.003ArticleGoogle Scholar
- Choobbasti AJ, Farrokhzad F, Barari A (2009) Prediction of slope stability using artificial neural network (case study: Noabad, Mazandaran, Iran). Arab J Geosci 2(4):311–319. https://doi.org/10.1007/s12517-009-0035-3ArticleCASGoogle Scholar
- Das SK, Biswal RK, Sivakugan N, Das B (2011) Classification of slopes and prediction of factor of safety using differential evolution neural networks. Environ Earth Sci 64:201–210. https://doi.org/10.1007/s12665-010-0839-1ArticleGoogle Scholar
- Dashbold B, Bryson LS, Crawford MM (2023) Landslide hazard and susceptibility maps derived from satellite and remote sensing data using limit equilibrium analysis and machine learning model. Nat Hazards 116(1):235–265. https://doi.org/10.1007/S11069-022-05671-7/METRICSArticleGoogle Scholar
- Demir S, Sahin EK (2023) Application of state-of-the-art machine learning algorithms for slope stability prediction by handling outliers of the dataset. Earth Sci Inf 16(3):2497–2509. https://doi.org/10.1007/s12145-023-01059-8ArticleGoogle Scholar
- Fellenius W (1936) Calculation of the stability of earth dams. Proc Second Congress Large Dams 4:445–463 Google Scholar
- Froude MJ, Petley DN (2018) Global fatal landslide occurrence from 2004 to 2016. Nat Hazards Earth Syst Sci 18(8):2161–2181. https://doi.org/10.5194/NHESS-18-2161-2018ArticleGoogle Scholar
- Gelisli K, Kaya T, Babacan AE (2015) Assessing the factor of safety using an artificial neural network: case studies on landslides in Giresun, Turkey. Environ Earth Sci 73:8639–8646. https://doi.org/10.1007/s12665-015-4027-1ArticleGoogle Scholar
- Gleason CJ, Im J (2012) Forest biomass estimation from airborne LiDAR data using machine learning approaches. Remote Sens Environ 125:80–91. https://doi.org/10.1016/j.rse.2012.07.006ArticleGoogle Scholar
- Haykin S (1999) Self-organizing maps. Neural Networks-A Comprehensive Foundation, 2nd Edition, Prentice-Hall
- He X, Li SJ, Liu YX, Zhou Y (2003) Analyzing method of rock slope stability based on artificial neural network. Rock Soil Mech 24(S1):73–76. CASGoogle Scholar
- Huang F, Chen J, Liu W, Huang J, Hong H, Chen W (2022) Regional rainfall-induced landslide hazard warning based on landslide susceptibility mapping and a critical rainfall threshold. Geomorphology 408:108236. https://doi.org/10.1016/j.geomorph.2022.108236ArticleGoogle Scholar
- Janbu N (1955) Application of composite slip surfaces for stability analysis. European Conferrence on Stability of Earth Slopes, 1955 3:43–49
- Juang CH, Lee DH, Sheu C (1992) Mapping slope failure potential using fuzzy sets. J Geotech Eng 118(3):475–494 ArticleGoogle Scholar
- Karir D, Ray A, Bharati AK, Chaturvedi U, Rai R, Khandelwal M (2022) Stability prediction of a natural and man-made slope using various machine learning algorithms. Transp Geotechnics 34:100745 ArticleGoogle Scholar
- Kasa H (1992) Study on landslide prediction model using satellite remote sensing data and geographic information. Proceedings of the 6th International Symposium on Landslides. 2:983–988
- Kavzoglu T, Colkesen I (2009) A kernel functions analysis for support vector machines for land cover classification. Int J Appl Earth Obs Geoinf 11(5):352–359 Google Scholar
- KC R, Aryal M, Dahal BK, Sharma K (2021) Spatial and temporal analysis of landslides during last decade in Nepal. In: Proceedings of 10th IOE Graduate Conference, pp 102–106. https://www.researchgate.net/publication/358987469_Spatial_and_Temporal_Analysis_of_Landslides_during_Last_Decade_in_Nepal
- KC R, Sharma K, Dahal BK et al (2024) Study of the spatial distribution and the temporal trend of landslide disasters that occurred in the Nepal Himalayas from 2011 to 2020. Environ Earth Sci 83:42. https://doi.org/10.1007/s12665-023-11347-7
- Koopialipoor M, Jahed Armaghani D, Hedayat A, Marto A, Gordan B (2019) Applying various hybrid intelligent systems to evaluate and predict slope stability under static and dynamic conditions. Soft Comput 23:5913–5929 ArticleGoogle Scholar
- Kurnaz TF, Erden C, Dağdeviren U, Demir AS, Kökçam AH (2024) Comparison of machine learning algorithms for slope stability prediction using an automated machine learning approach. Nat Hazards 120(8):6991–7014. https://doi.org/10.1007/s11069-024-06490-8ArticleGoogle Scholar
- Lehtonen V (2015) Modelling undrained shear strength and pore pressure based on an effective stress soil model in Limit Equilibrium Method.
- Lin Y, Zhou K, Li J (2018) Prediction of slope stability using four supervised learning methods. IEEE Access 6:31169–31179. https://doi.org/10.1109/ACCESS.2018.2843787ArticleGoogle Scholar
- Lin S, Zheng H, Han C, Han B, Li W (2021) Evaluation and prediction of slope stability using machine learning approaches. Front Struct Civil Eng 15(4):821–833 ArticleGoogle Scholar
- Liu Z, Gilbert G, Cepeda JM, Lysdahl AOK, Piciullo L, Hefre H, Lacasse S (2021) Modelling of shallow landslides with machine learning algorithms. Geosci Front 12(1):385–393 ArticleCASGoogle Scholar
- McAdoo BG, Quak M, Gnyawali KR, Adhikari BR, Devkota S, Rajbhandari L, P., Sudmeier-Rieux K (2018) Roads and landslides in Nepal: how development affects environmental risk. Nat Hazards Earth Syst Sci 18(12):3203–3210. https://doi.org/10.5194/nhess-18-3203-2018ArticleGoogle Scholar
- Melchiorre C, Matteucci M, Azzoni A, Zanchi A (2008) Artificial neural networks and cluster analysis in landslide susceptibility zonation. Geomorphology 94(3–4):379–400. https://doi.org/10.1016/j.geomorph.2006.10.035ArticleGoogle Scholar
- Morgenstern NR u, Price VE (1965) The analysis of the stability of general slip surfaces. Geotechnique 15(1):79–93. https://doi.org/10.1680/geot.1968.18.3.393
- Nasseri MHB, Mohanty B, Esmaeili K, Kolapo P, Omotayo Oniyide G, Said KO, Lawal AI, Onifade M, Munemo P (2022) An overview of Slope failure in Mining Operations. Min 2022 2(2):350–384. https://doi.org/10.3390/MINING20200192ArticleGoogle Scholar
- Paudyal P, Dahal P, Bhandari P, Dahal BK (2023) Sustainable rural infrastructure: guidelines for roadside slope excavation. Geoenvironmental Disasters 10(1):11. https://doi.org/10.1186/s40677-023-00240-xArticleGoogle Scholar
- Pradhan B, Lee S, Buchroithner MF (2010) A GIS-based back-propagation neural network model and its cross-application and validation for landslide susceptibility analyses. Comput Environ Urban Syst 34(3):216–235. https://doi.org/10.1016/j.compenvurbsys.2009.12.004ArticleGoogle Scholar
- Pyakurel A, Dahal BK, Gautam D (2023) Does machine learning adequately predict earthquake induced landslides? Soil Dyn Earthq Eng 171:107994. https://doi.org/10.1016/j.soildyn.2023.107994ArticleGoogle Scholar
- Qi C, Tang X (2018) Slope stability prediction using integrated metaheuristic and machine learning approaches: a comparative study. Comput Ind Eng 118:112–122 ArticleGoogle Scholar
- Ray A, Kumar V, Kumar A, Rai R, Khandelwal M, Singh TN (2020) Stability prediction of himalayan residual soil slope using artificial neural network. Nat Hazards 103:3523–3540. https://doi.org/10.1007/s11069-020-04141-2ArticleGoogle Scholar
- Samui P (2008) Slope stability analysis: a support vector machine approach. Environ Geol 56(2):255–267. https://doi.org/10.1007/s00254-007-1161-4ArticleGoogle Scholar
- Sim K, Ben, Lee ML, Wong SY (2022) A review of landslide acceptable risk and tolerable risk. Geoenvironmental Disasters 9(1):1–17. https://doi.org/10.1186/S40677-022-00205-6/FIGURES/10ArticleGoogle Scholar
- Spencer E (1967) A method of analysis of the stability of embankments assuming parallel inter-slice forces. Geotechnique 17(1):11–26. https://doi.org/10.1680/geot.1967.17.1.11ArticleGoogle Scholar
- Subedi M, KC R, Acharya IP (2021) SPT-based seismic soil liquefaction potential evaluation of Kathmandu Valley using first-order second-moment method. In Proceedings of the GeoNiagara Conference 2021, Niagara Falls, ON, Canada, 26–29 September
- Subedi M, KC R, Sharma K, Misra J, KC A (2024) Reconnaissance of the effects of the MW5.7 (ML6.4) Jajarkot Nepal Earthquake of 3 November 2023, post-earthquake responses, and Associated lessons to be learned. Geosciences 14:20. https://doi.org/10.3390/geosciences14010020ArticleGoogle Scholar
- Thakur AS, Pandya S, Sachan A (2021) Dynamic behavior and characteristic failure response of low plasticity cohesive soil. Int J Civil Eng 19(2):167–185. https://doi.org/10.1007/s40999-020-00560-1ArticleGoogle Scholar
- Tien Bui D, Moayedi H, Gör M, Jaafari A, Foong LK (2019) Predicting slope stability failure through machine learning paradigms. ISPRS Int J Geo-Information 8(9):395. https://doi.org/10.3390/ijgi8090395ArticleGoogle Scholar
- Wang H, Bin, Sassa K (2006) Rainfall-induced landslide hazard assessment using artificial neural networks. Earth Surf Proc Land 31(2):235–247. https://doi.org/10.1002/ESP.1236ArticleGoogle Scholar
- Wongvibulsin S, Wu KC, Zeger SL (2020) Clinical risk prediction with random forests for survival, longitudinal, and multivariate (RF-SLAM) data analysis. BMC Med Res Methodol 20(1):1–14 ArticleGoogle Scholar
- Ye P, Yu B, Chen W, Liu K, Ye L (2022) Rainfall-induced landslide susceptibility mapping using machine learning algorithms and comparison of their performance in Hilly area of Fujian Province, China. Nat Hazards 2022 113:2(2):965–995. https://doi.org/10.1007/S11069-022-05332-9. 113 ArticleGoogle Scholar
- Yilmaz I (2010) The effect of the sampling strategies on the landslide susceptibility mapping by conditional probability and artificial neural networks. Environ Earth Sci 60:505–519. https://doi.org/10.1007/s12665-009-0191-5ArticleGoogle Scholar
- Zare M, Pourghasemi HR, Vafakhah M, Pradhan B (2013) Landslide susceptibility mapping at Vaz Watershed (Iran) using an artificial neural network model: a comparison between multilayer perceptron (MLP) and radial basic function (RBF) algorithms. Arab J Geosci 6:2873–2888. https://doi.org/10.1007/s12517-012-0610-xArticleGoogle Scholar
- Zou Q, Jiang H, Cui P, Zhou B, Jiang Y, Qin M, Liu Y, Li C (2021) A new approach to assess landslide susceptibility based on slope failure mechanisms. CATENA 204:105388. https://doi.org/10.1016/J.CATENA.2021.105388ArticleGoogle Scholar
Funding
The authors did not receive any funding for this research.